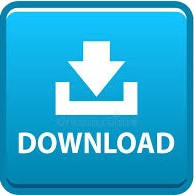
Wald Estimator and Two-Stage Least Squares Estimator: From the Sesame Street Example Monotonicity: Assume that there were no children who would watch if they were not encouraged but who would not watch if they were encouraged (no defiers)Įxclusion restriction: The instrument has no direct effect on the outcome, except indirectly through the treatment Nonzero associaiton between IV and treatment variable: The instrument must have an effect on the treatment Ignorability of the instrument: The instrument should be randomized or conditionally randomized with respect to the outcome and treatment variables
#Equation systems for variables regression trial
Taking advantage of the randomization of encouragement, could esitmate a causal effect of watching for at least some of the people in the studyĪs shown above in the below, the children in the trial could be categorized according to their compliance statusĬompliers are the only children for whom we will make inferences about the effect of watching Sesame Street and this effect is referred as the Complier Average Causal Effect (CACE) In this experiment, watching itself cannot be randoimzed but only encouragement to watch the show can be randomly assigned
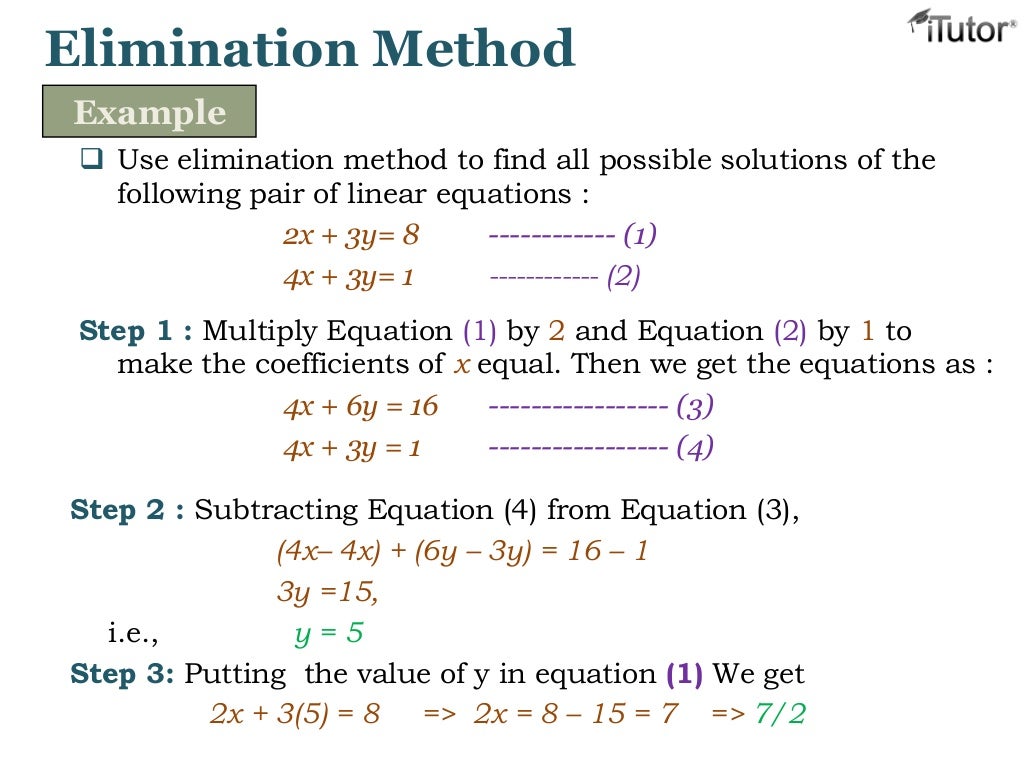
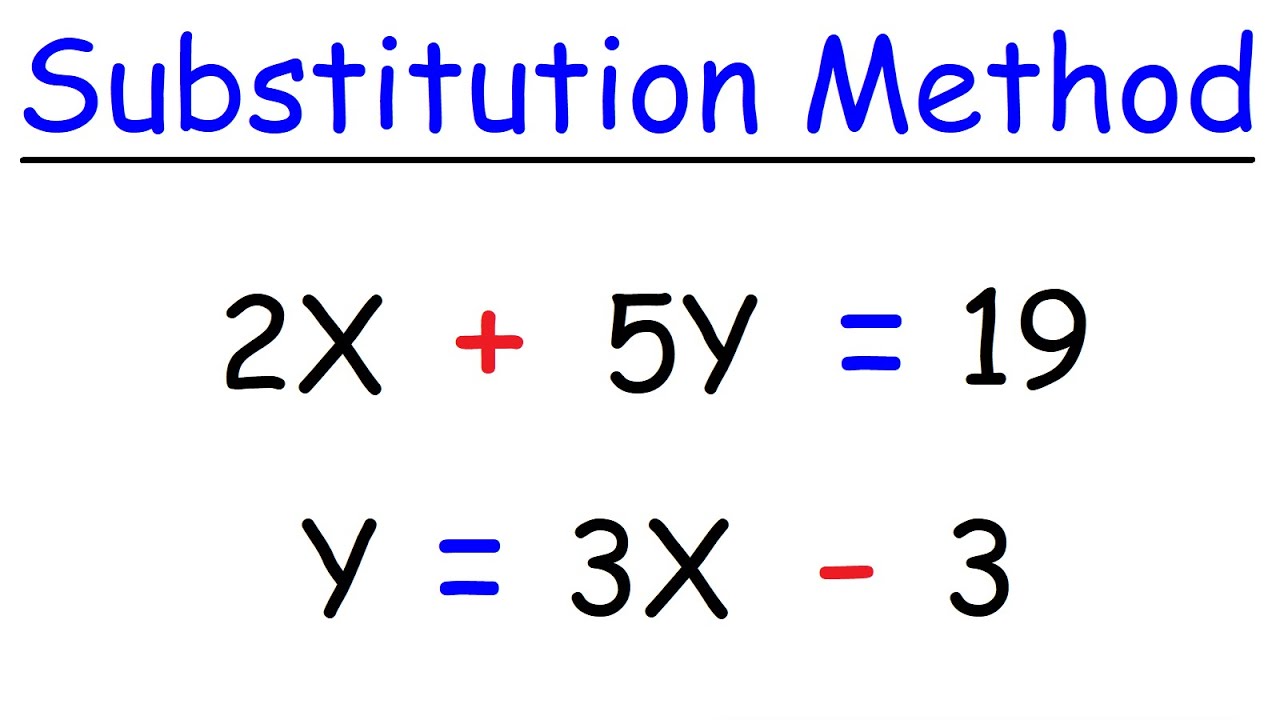
#Equation systems for variables regression tv
If we assume a situation where an experimenter implemented a randomized experiment where the participants are preschool children, in which the treatment is Watching Sesame Street TV Program, and the outcome of interest is score on letter recognition test The bias can be large in the case of weak instrumentsĬompliance Status from Potential Outcome Framework when we have so-called ‘weak instruments’) and only has large sample properties (consistency) IV is not as efficient as OLS (especially if Z only weakly correlated with X, i.e. With this new variable, the IV estimator should capture only the effects on Y of shifts in Xinduced by whereas the OLS estimator captures not only the direct effect of on but also the effect of the included measurement error and/or endogeneity In the above example z2 and z1 are straightforward instruments for IV estimation of the first and second equation, respectively.Īn instrumental variable, Z is uncorrelated with the disturbance e but is correlated with X(e.g., proximity to college might be correlated with schooling but not with wage residuals) Online appendix.The above equation system is also described as reversed causality because the dependent variable y1 has a feedback effect on the regressor y2. "Normal equations", Lectures on probability theory and mathematical statistics. If you want to double check the formulae and the derivations shown above, you More mathematical details about the normal equations and the OLS estimator can Therefore, we can employ the standard methods for solving linear systems.įor example, if the equations are expressed in matrix form and the matrix That solves the system is the OLS estimator of the vectorĪs stated above, the normal equations are just a system of If the system has a unique solution, the value of Thus, in the case of the multiple regression model, the normal equations,Įxpressed above in matrix form, are a system of Is a system of normal equations expressed in matrix form. (the vector of partial derivatives with respect to the entries of
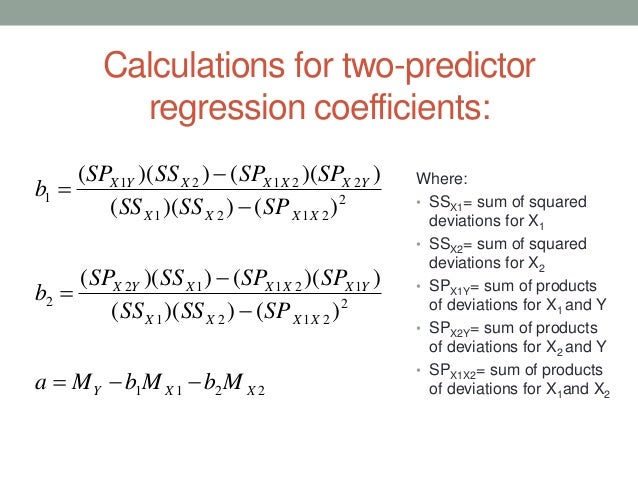
Order to find a minimizer, we compute the first-order condition for a minimum. Is derived by minimizing the sum of squared residuals, which can be written in The normal equations for the multiple regression model are expressed in Normal equations in the multiple regression model Matrix of regressors (the so-called design The regression equation can be written in matrix In a multiple linear regression, in which there is more than one regressor, That solve the system are the OLS estimators of the intercept Thus, in the case of a simple linear regression, the normal equations are a
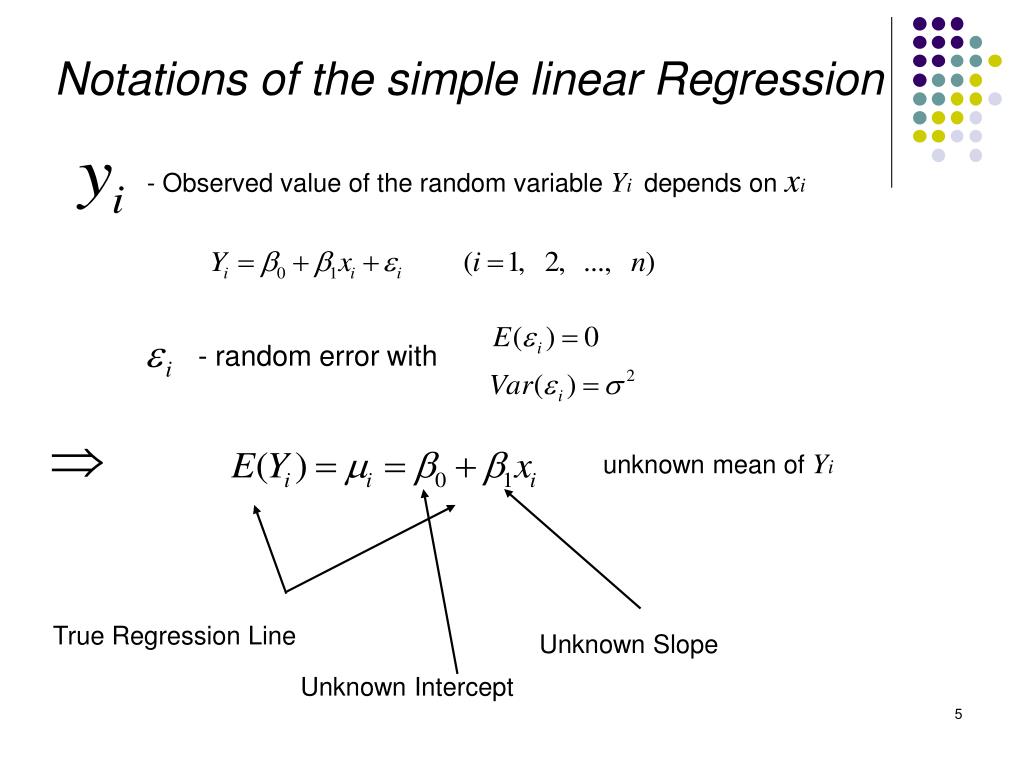
In other words, we calculate the derivatives ofĪre the two normal equations displayed above. Are derived by minimizing the sum of squaredĬarry out the minimization by computing the first-order conditions for a
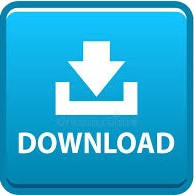